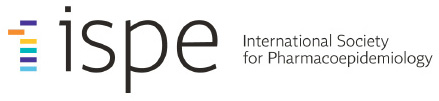
ICPE conference
A Decade of Experience With High-Dimensional Propensity Scores
-
Register
- Non-Members - Free!
- Members - Free!
Speaker: Sebastian Schneeweiss, MD, FISPE, Brigham and Women's Hospital (Division of Pharmacoepidemiology and Pharmacoeconomics)
Longitudinal healthcare claims data can be viewed and analyzed as a set of proxies that indirectly describe the health status of patients. This status is presented through the lenses of health care providers recording their findings and interventions via coders and operating under the constraints of a specific health care system. While direct measures of potentially important confounders may not be readily available in claims, batteries of proxies could act as surrogates for these otherwise unmeasured variables. For example, frailty is not directly measured, but codes indicating use of oxygen canisters, wheelchairs, walkers, and commode chairs may be indicators of frailty. Adjusting for these surrogates will adjust for an unobserved or imperfectly measured confounder proportional to the degree to which the proxies and confounders are correlated.
Using the idea of proxy adjustment, the high-dimensional propensity score (hd-PS) algorithm identifies a large number of covariates in claims databases, eliminates covariates with very low prevalence and minimal potential for causing bias, and then uses propensity score techniques to adjust for a large number of target covariates. The use of hd-PS is increasing in pharmacoepidemiologic studies.